A can of worms: the non-significant effect of deworming on happiness
- A can of worms: the non-significant effect of deworming on happiness
- Summary
- 1. Background and literature
- 2. Wellbeing analysis
- 3. Cost-effectiveness analyses
- 4. Our recommendation for donors
- 5. Limitations
- 6. Future research to address limitations
- 7. Conclusion
Summary
Mass deworming, where many people are provided drugs to treat parasitic worms, has long been considered a highly cost-effective intervention to improve lives in low-income countries. GiveWell directed over $163 million to deworming charities since 2010. Nevertheless, there are long-running debates about its impact and cost-effectiveness. In this report, we summarise the debate about the efficacy of deworming, present the first analysis of deworming in terms of subjective wellbeing (SWB), and compare the cost-effectiveness of deworming to StrongMinds (our current top recommended charity).
Analysing SWB data from the Kenyan Life Panel Survey (KLPS; Hamory et al., 2021), we find that deworming has a small, statistically non-significant effect on long-term happiness that seems (surprisingly) to become negative over time (see Figure 1). We conclude that the effect of deworming in the KLPS is either non-existent or too small to estimate with certainty. Typically, an academic analysis could stop here and not recommend deworming. However, the non-significant effects of deworming could be cost-effective in practice because it is extremely cheap to deliver. Because the effect of deworming is small and becomes negative over time, our best guess finds that the overall cost-effectiveness of deworming is negative. Even under more generous assumptions (but still plausible according to this data), deworming is less cost-effective than StrongMinds. Therefore, we do not recommend any deworming charities at this time. To overturn this conclusion, proponents of deworming would either need to (1) appeal to different SWB data (we’re not aware of any) or (2) appeal to a non-SWB method of comparison which concludes that deworming is more cost-effective than StrongMinds.
Figure 1: Differences in happiness between treatment and control groups over time
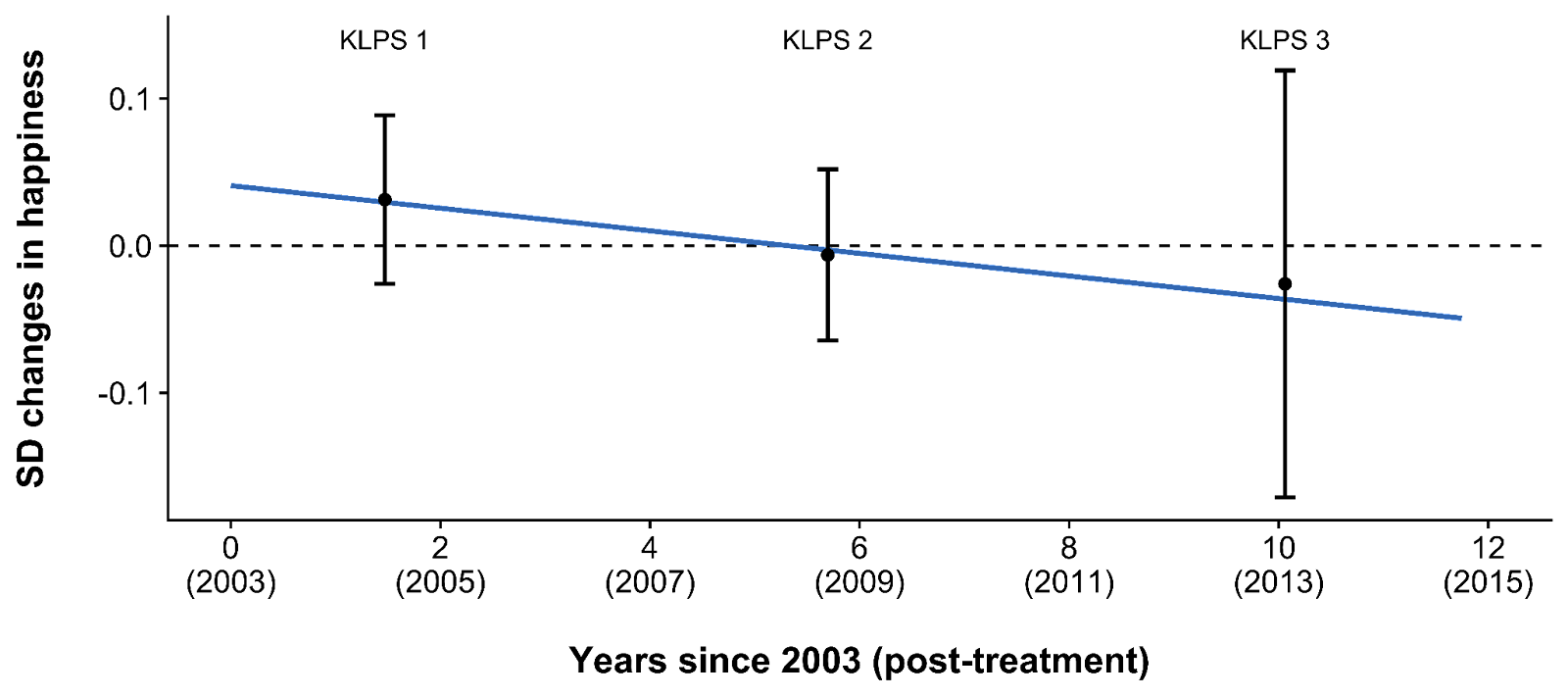
Note. The point estimates show the difference (in Cohen’s d) in happiness between the treatment and control group at each time point. The bars represent 95% confidence intervals. The regression line shows the trend of the difference between treatment and control over time.
1. Background and literature
In this section, we present the motivation for this analysis, the work by GiveWell that preceded this, and the broader literature on deworming. We then present the details and context of the dataset we use for this analysis – the Kenyan Life Panel Survey (KLPS).
1.1 Our motivation for this analysis
The Happier Lives Institute evaluates charities and interventions in terms of subjective wellbeing (SWB) – how people think and feel about their lives. We believe that wellbeing is what ultimately matters and we take self-reports of SWB to be the best indicator of how much good an intervention does. If deworming improves people’s lives, those treated for deworming should report greater SWB than those who aren’t. SWB should capture and integrate the overall benefits from all of the instrumental goods provided by an intervention.1Note that a large body of research shows that changes to one’s life circumstances are reflected in people’s self-reports (Clark et al., 2018; Dolan et al., 2008; Kahneman & Krueger, 2006; Kaiser & Oswald, 2022). Hence, to see if something works, we can often just ‘short-cut’ straight to the subjective wellbeing data, rather than looking at other instrumental outcomes (e.g., economic benefits). For example, if deworming makes people richer, and this makes them happier, they will report higher SWB (the same is true for improvements to health or education). Although we are not the first to use SWB as an outcome for decision-making (e.g., UK Treasury, Frijters et al., 2020, Birkjaer et al., 2020, Layard & Oparina, 2021), we are the first to use it to compare the impact of charities. See McGuire et al. (2022b) for more detail about why we prefer the SWB approach to evaluate charities.
To determine whether the SWB approach changes which interventions we find the most cost-effective, we have been re-evaluating the charity recommendations of GiveWell (a prominent charity evaluator that recommends charities based on their mortality and economic impacts). For a review of our recent research, see this post. We present our findings in wellbeing-adjusted years (WELLBYs), where 1 WELLBY is the equivalent of a 1-point change on a 0-10 SWB measure.
1.2 GiveWell’s history with deworming
From 2010 until August 2022, GiveWell’s list of top charities included four charities that provide mass deworming.2SCI Foundation, Evidence Action’s Deworm the World Initiative, Sightsavers’s deworming program, and The END Fund’s deworming program. However, deworming does not fulfil their new criteria for top charities because it does not have “a high likelihood of substantial impact”. Although GiveWell “no longer accept donations on behalf of […] the four deworming programs previously on our top charity list”, they still provide funding to these charities through their All Grants Fund.
GiveWell’s (2018) analysis of the long-term effects of deworming is based on a single dataset, the Kenyan Life Panel Survey (KLPS; Hamory et al., 2021), which follows recipients from a deworming program in Kenya (see Section 1.3.3 for more details). They treat the primary benefits of deworming as the relative income and consumption benefits accumulated in the 20 years following treatment (Hamory et al., 2021). GiveWell has investigated deworming for much longer than we have and we rely on some of their work (e.g., worm burden adjustments and charity costs) in our analysis. Nevertheless, we aim to improve on this earlier analysis in two ways:
- Adequately accounting for total effects over time. GiveWell focuses on economic effects and assumes that these do not decay over time. In an earlier reanalysis of the economic effects, we pointed out that this was not justified by the relevant data: the KLPS study shows a decay over time, which substantially reduces the total effects (see our detailed critique).
- Measuring the effects of deworming on SWB, not wealth. Contrary to GiveWell, we make use of the SWB data available in the KLPS. As noted, we believe ours is the first attempt to assess the cost-effectiveness of deworming in terms of SWB.
1.3 What is deworming and what are the arguments over its effectiveness?
Parasitic infections from worms affect around a billion people in mostly low- and middle-income countries (see Figure 2), and cause a range of health problems (Else et al., 2020; WHO, 2011). Lack of proper sanitation or health behaviours increases the risk of transmission because infected individuals can contaminate soil and water via their waste. These infections can cause a range of urinary, intestinal, nutritional, cognitive, and developmental problems.
Figure 2: Overview of parasitic worm types targeted by deworming and their global prevalence
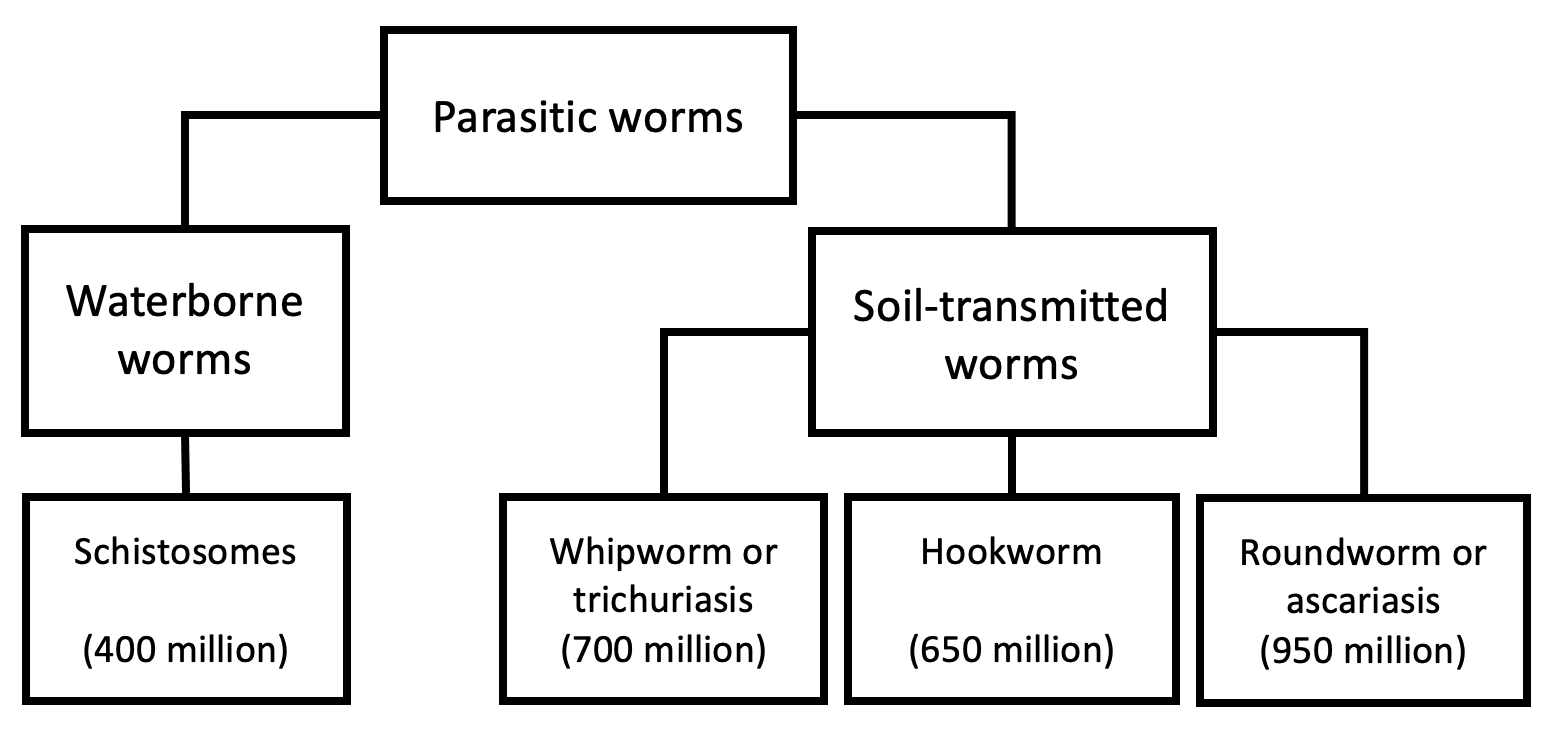
Note. This description and data is adapted from GiveWell’s (2018) report on deworming.
The World Health Organisation (WHO) recommends mass deworming as treatment for parasitic worms: providing anti-parasite drugs to a general (unscreened) population, usually school children, to control the prevalence of worms in an area (Else et al., 2020; WHO, 2006, 2011, 2020). Testing for worm infection is expensive but the drugs are cheap and unlikely to cause side effects3Although, see GiveWell’s section on the potential negative effects of mass deworming. so they are provided to individuals without prior testing. Mass deworming decreases worm loads (e.g., Danso‐Appiah et al., 2008), although the efficacy depends on the type of parasitic worm (Else et al., 2020). Despite the general success of deworming at removing the parasites, evidence of improvements to general health or other benefits is weak.
1.3.1 The worm wars: short-term RCT evidence of deworming
The short-term effects of deworming on children’s health, cognition, and education are subject to a long and ongoing academic controversy related to the quality of its evidence bases and the significance of its results. The core of the debate centres around a series of meta-analyses of randomised controlled trials (RCTs).
Taylor-Robinson et al. (first version: 2012, latest version: 2019) argue in a Cochrane review that:
[deworming does] not appear to improve height, haemoglobin, cognition, school performance, or mortality. We do not know if there is an effect on school attendance, since the evidence is inconsistent and at risk of bias, and there is insufficient data on physical fitness. Studies conducted in two settings over 20 years ago showed large effects on weight gain, but this is not a finding in more recent, larger studies. We would caution against selecting only the evidence from these older studies as a rationale for contemporary mass treatment programmes as this ignores the recent studies that have not shown benefit.
Welch et al. (2016, 2019), in a Campbell collaboration, replicate and concur with the Cochrane review. In a meta-analysis focused on mortality, anaemia4They also did not find an effect of deworming on anaemia for women in their meta-analysis. In slight contrast, Salam et al.’s (2019) individual participant level meta-analysis found that deworming significantly reduces anaemia in pregnant women, but does not affect birthweight or likelihood of preterm birth for their children., and growth for children, Thayer et al. (2017) finds similarly inconsistent benefits. A meta-analysis focusing on the cognitive effects of deworming (Pabalan et al., 2018) echoed the null results of the Campbell and Cochrane reviews. In most cases, this would be the last word on the issue as Cochrane and Campbell are often regarded as the gold standard for systematic reviews across many fields. And it probably would have been – if it weren’t for the recent work of Croke and colleagues.
Croke et al. (first version: 2016, latest version: 2022), a team of economists including a Nobel laureate, meta-analysed the literature on deworming using a broader set of studies and different statistical techniques. They find – contrary to the Cochrane and Campbell reviews – that deworming has a small but statistically significant effect on weight, thus reopening the debate about the potential benefits of deworming.5For simplicity, we do not delve into the details of this debate, which centre around nuanced methodological details that are not relevant for this report. The key point is that the effects of deworming are debated among experts.
Proponents of deworming argue that even if the effects of deworming are small, it may still be cost-effective because deworming can be incredibly cheap ($1 per person per year of treatment according to GiveWell’s analysis). Taking Croke et al.’s figures at face value, Ahuja et al. (2018) calculated that deworming is 40 times more cost-effective at increasing children’s weight than standard school food programmes.
If we rely solely on evidence from RCTs on short-term effects, then there are no consistently detectable effects, and the evidence is debated amongst experts. However, there are quasi-experimental and experimental studies of long-term effects to consider.
1.3.2 Long-term quasi-experimental evidence of deworming
The meta-analyses discussed above only considered RCTs of short-term outcomes. We found three (there may be more) historical quasi-experimental studies that also attempt to measure the causal impact of deworming. These studies examine the long-term impact of permanent deworming ‘eradication’ – instead of yearly deworming ‘control’ – in eras and contexts that are different to those where mass deworming is commonly deployed. These studies cover deworming eradication campaigns in1920s USA (Bleakley, 2007), 1950s China (Liu & Liu, 2019), and early 2000s Nigeria (Makamu et al., 2018). These natural experiments have a combined sample size of more than a million participants (comparable to all of the RCTs combined). They find more precise and more consistently positive outcomes for the long-term effects on income (Liu & Liu, 2019; Bleakley, 2007), education (Liu & Liu, 2019; Bleakley, 2007; Makamu et al., 2018), and cognition (Liu & Liu, 2019).
If we include quasi-experiments of deworming eradication, deworming appears more promising. However, we are unsure how relevant this historical evidence is to current deworming programmes which have pursued a ‘control’ rather than ‘elimination’ strategy, operated in different countries, and operated in environments with different worm burdens. For someone to argue that these studies generalise to the modern context of today’s deworming charities, they need to demonstrate that the features that differ between contexts aren’t relevant to the outcomes of interest.6We touch on some of the difficulties with extrapolating from historical quasi-experiments to modern intervention contexts in a recent essay (McGuire et al., 2022d). Vetting the sensibility of this extrapolation ourselves – and converting these effects into SWB effects – is beyond the scope of this report. Because historical deworming eradication and modern mass deworming campaigns differ substantially, the quasi-experimental evidence only weakly updates our views. However, the main data used for cost-effectiveness analyses of deworming is the KLPS, which is more relevant to today’s charities, and which we present below.
1.3.3 Long-term experimental evidence: the KLPS data
Experimental evidence of the long-term effects of interventions that aim to control (instead of eradicate) the burden of parasitic worms comes primarily from one study: the Kenya Life Panel Survey (KLPS; Baird et al., 2016; Hamory et al., 2021).7See Jullien et al. (2017) for a review of long-term effects. The KLPS follows a subset of participants from one deworming program, the Primary School Deworming Project (PSDP; Miguel & Kremer, 2004). GiveWell’s (2018, 2022) estimate of the effect of deworming primarily relies on the long-term earnings and consumption gains observed from this study. Since the KLPS is the sole source of evidence on economic effects that GiveWell uses, and the sole source of SWB data we could find, we explain the study and its follow-up data in detail below.
The PSDP was implemented in southern Busia in Kenya by Internationaal Christelijk Steunfonds Africa (a Dutch NGO) and the Busia District Ministry of Health office. They pseudo-randomised 75 schools (32,565 pupils) into three groups of 25 which received deworming8On average, children would receive 2.25 deworming pills in a year of treatment for both soil- and water-transmitted worms (depending on local prevalence). This is not accounting for the fact that uptake was not exactly 100% for treatment and 0% for control in a year where they are not supposed to receive treatment. Indeed, there was about 75% uptake of some medication (at least one) for the treatment in a given year and 5% for the control (Baird et al., 2016; Miguel & Kremer, 2004). and health education9Students in the treatment group also received health education, which involved “regular public health lectures, wall charts and training of teachers” (p. 169, Miguel & Kremer 2004). It emphasised hand washing, wearing shoes, and avoiding swimming in freshwater. An obvious concern is that any effects we see could be partially or completely driven by the health education, not the deworming medication. Miguel and Kremer (2004; Table 5) argue, in our view convincingly, that this was not the case because there was zero difference in observed and self-reported health behaviours between conditions. starting at different times across 1998-2003. Group 1 received ~6 years of deworming treatment (starting in 1998), Group 2 received ~5 years of deworming treatment (starting in 1999), and Group 3 received ~3 years of deworming treatment (starting in 2001). Hence, Groups 1 and 2 received, on average, 2.41 extra years of deworming than Group 3. Groups 1 and 2 are considered ‘treatment’ groups (50 schools) and Group 3 is considered a ‘control’ group (25 schools). Hence, when we mention ‘the effect of deworming’ we are really talking about ‘the effect of more deworming’. Although there is no ‘true’ control who did not receive deworming, the existing control group should provide a conservative reference group.
The data comes from the KLPS which follows a sample of ~7,500 PSDP participants with tracking rates of ~84% from 2003-2019 (Baird et al., 2016; Hamory et al., 2021). There are four rounds of the KLPS: KLPS 1 (2003-2005), KLPS 2 (2007-2009), KLPS 3 (2011-2014), and KLPS 4 (2017-2019). Rounds 1-3 are available online. KLPS 4 is not available online yet, but replication materials for Hamory et al.’s (2021) economic analyses of that dataset are available online.
In early surveys of PSDP recipients, Miguel and Kremer (2004) found that deworming significantly increased school participation in 1999 (but not test scores or weight, nor reduced anaemia).10The analysis by Miguel and Kremer (2004) has been the object of multiple replications and debates – including a correction to the anaemia result which was first reported as significant (Humphreys, 2015; Miguel et al., 2015). Replication works include, amongst others: Aiken et al. (2015), Davey et al. (2015), and Humphreys (2015). See the authors reply (Miguel et al., 2015) and GiveWell’s (2015) summary for more details. We use the KLPS, which is not the data Miguel and Kremer analyse, so this does not affect our analysis. Baird et al. (2016) and Hamory et al. (2021) focus on the long-term benefits of deworming using the KLPS. Note that the previously discussed meta-analyses focused on the short-term effects of deworming, so they included findings from Miguel and Kremer but not from Baird et al. and Hamory et al.11Baird et al. (2016) was excluded from the Cochrane (2019) review because it was deemed “at risk of substantial methodological bias” (p. 14). Hamory et al. (2021) came out after the Cochrane review. At the 10-year follow-up (KLPS 2), Baird et al. (2016) found that deworming significantly increased economic and educational outcomes for some subsets of the population. Hamory et al. (2021) found that deworming produced a non-significant increase in earnings and consumption from the 10-year to the 20-year follow-up (KLPS 2 to KLPS 4). GiveWell’s analysis is based on the estimated relative earnings and consumption benefits for recipients of deworming. GiveWell (2016, 2018) argues that deworming is likely to be cost-effective on the grounds that, even after discounting the effect by almost 99%, the effect still suggests that deworming has high (albeit uncertain) expected value in terms of economic benefits.
Before our analysis of the wellbeing data, we present potential pathways through which deworming can improve wellbeing in the section below.
1.3.4 Potential causal pathways
In Figure 3, we illustrate a simple model of the potential pathways for deworming to influence wellbeing (inspired by Taylor-Robinson et al., 2019). To give one example of the many pathways, deworming in childhood may lead to improved cognitive abilities, which increases education, which in turn yields a greater income later in life, which ultimately benefits wellbeing. But again, we think the evidence that deworming impacts any of these outcomes is uncertain.
Figure 3: Diagram of causal mechanisms for deworming to impact wellbeing
2. Wellbeing analysis
In this section, we present our modelling of the impact of deworming on wellbeing. In Section 2.1 we present the wellbeing data we use. In Section 2.2 we present our non-significant results for the effect of deworming on happiness. In Section 2.3 we interpret these non-significant results.
2.1 The KLPS wellbeing data
Although there are multiple measures of SWB in the KLPS data, only one measure (hereafter, happy123) was included across all three available follow-ups.12Other measures available include a 1-10 happiness measure for a sub-sample of KLPS 2, 1-6 frequency measures of different affective states for KLPS 3, and a range of binary measures of affective states. happy123 asked respondents: “Taking everything together, would you say you are somewhat happy, very happy or not happy? (very happy, somewhat happy, not happy, don’t know)”.13We treat “don’t know” responses as missing and reverse-coded the data so that 1 = not happy, 2 = somewhat happy, and 3 = very happy. In Appendix A3.1, we conduct a version of this analysis where we combine all the available measures and find similar results, which suggests that the analysis using happy123 is consistent with the other measures.
In Table 1, we show how many responses were provided for each condition. Note that the KLPS tracks 7,527 respondents – 2,564 in the control condition and 4,963 in the treatment condition.14Fewer than 7,527 participants respond at each round because of attrition. In KLPS 3, the researchers only administered happy123 to a subset of participants. We do not think this is problematic because (1) the user guide for the data mentions that the subset (1,312 individuals) was designed to be representative of the rest of the sample, and (2) whilst this makes for a smaller sample at KLPS 3, we use a meta-analysis which will weight data from KLPS 3 less because of this loss in precision.
Table 1: Number of responses to happy123 across the follow-ups
KLPS round | |||
---|---|---|---|
1 |
2 |
3 |
|
Respondents to happy123 in the control condition |
1,783 |
1,707 |
276 |
Respondents to happy123 in the treatment condition |
3,417 |
3,380 |
539 |
Total respondents to happy123 |
5,200 |
5,087 |
815 |
Total respondents in data |
5,209 |
5,094 |
5,259 |
Note. The response rate to happy123 was 69% at KLPS 1, 68% at KLPS 2, and 62% at KLPS 3. The response rates were nearly identical in the treatment and control groups at each time point, suggesting that differential attrition by group was not an issue in the study.
2.2 Non-significant effects of deworming on happiness
The goal of our primary analysis is to calculate the total effect of deworming on wellbeing over time. To do so, we standardise the mean difference in happiness between the control and the treatment group with Cohen’s d (Lakens, 2013). We do this for each follow-up round of the KLPS. Then we use a meta-regression15A meta-analysis standardises and averages multiple effect sizes (across studies usually, but also across measures and follow-ups). Meta-regressions are a special form of meta-analysis and regression that explain how the effect sizes vary according to characteristics related to the effect sizes. Meta-regressions differ in a few technical ways from regressions that do not affect its interpretation. Typically, we use a meta-regression to summarise and explain effect sizes across multiple studies and time points (e.g., McGuire et al., 2022a). In our case, we use a meta-regression on the KLPS data – a single study with three effect sizes over time – where we explain the effect on happiness across multiple time points since treatment ended. See Harrer et al. (2021), for more detail on meta-regressions. to estimate the trajectory of the effect over time. We present a summary of the data we use for the meta-regression in Table 2.
Table 2: Summary of happy123 data used in the meta-regression
KLPS round |
Years since 2003 |
Control Mean (SD) |
Treatment Mean (SD) |
Mean difference |
SD pooled |
SE of d |
Cohen’s d |
---|---|---|---|---|---|---|---|
1 |
1.47 |
2.59 (0.62) |
2.61 (0.60) |
0.02 |
0.60 |
0.03 |
0.03 |
2 |
5.70 |
2.68 (0.52) |
2.68 (0.52) |
-0.0016-0.003 |
0.52 |
0.03 |
-0.01 |
3 |
10.06 |
2.44 (0.58) |
2.43 (0.60) |
-0.02 |
0.59 |
0.07 |
-0.03 |
Note. The SD pooled is the standard deviation for the mean difference. It is used to calculate Cohen’s d. The standard error (SE) of d is the error around Cohen’s d which is used for the 95 CI interval and to determine statistical significance.
To model the effects over time we need to define two parameters: the initial effect and the rate at which the effect decays. In our previous analyses (McGuire et al., 2022a), we’ve defined the initial effect as the effect when the treatment ends. In this case, both the treatment and the control conditions finish receiving their deworming treatment in 2003, which is also when the KLPS 1 data collection starts.
To determine the decay rate, we estimate the average difference in happiness across each KLPS round at their average follow-up in years since 2003. The earliest follow-up responses are on average 1.47 years after treatment ended (the middle of 2004).17The earliest individual response with a happy123 outcome comes from August 2003. Hence, the trendline for the decay is being extrapolated backwards a year and a half to estimate the initial effect. This is the same approach we took in our cost-effectiveness analysis of cash transfers (McGuire & Plant, 2021a). As we explain in Section 3, the total effect is very sensitive to exactly when we specify that the effects start and end.
The outcome of our model is reported in Table 3 and illustrated in Figure 4. The intercept is the initial effect (the effect post-treatment). The decay is how much the effect changes each year. According to this model, participants in the treatment group reported being 0.041 SDs happier than participants in the control group right after treatment, and this difference decayed by -0.008 SDs each year. The regression line predicts that the effect reaches zero and turns negative after 0.041 / 0.008 = 5.3 years. However, none of these effects are statistically significant (i.e., they are not distinguishable from 0) and the effects after 6 years (KLPS 2) and 10 years (KLPS 3) are – if anything – negative, so it is unclear if any of these effects are meaningful or just statistical noise. We discuss this in detail in the next section.
Table 3: Results of the meta-regression showing treatment effect on happiness over time
Term |
Estimate (in SDs) |
Standard Error |
t-value |
p-value |
---|---|---|---|---|
Intercept (‘initial effect’) |
0.041 |
0.036 |
1.121 |
.463 |
Years since 2003 (‘decay’) |
-0.008 |
0.008 |
-1.015 |
.495 |
Figure 4: Differences in happiness between treatment and control groups over time (repeated)
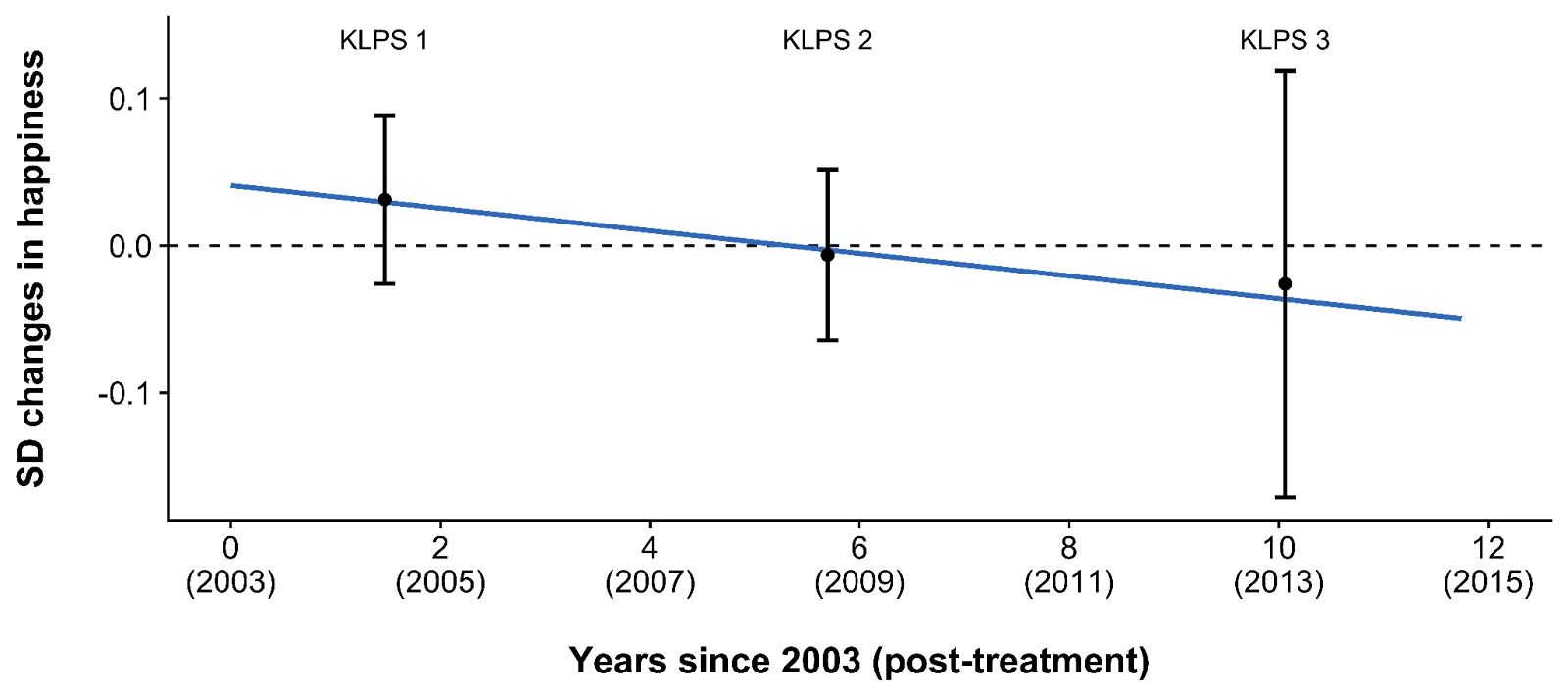
Note. The point estimates show the difference (in Cohen’s d) in happiness between the treatment and control group at each time point. The bars represent 95% confidence intervals. The regression line shows the trend of the differences between treatment and control over time.
2.3. Interpreting the results and non-significant effects
Although the effects of deworming were not significant at any point in our analysis, a statistically non-significant effect does not prove that the effect is zero; instead, it suggests that the effect is not estimated precisely enough to distinguish it from zero (for more detail see Goodman, 2008; Greenland et al., 2016). However, we have converging reasons to believe that in the present KLPS data there is no meaningful effect of deworming on long-term SWB.
- The happiness effects are small, non-significant, and distributed around zero (e.g., the effect in KLPS 1 is positive but negative at KLPS 2 and KLPS 3). Similarly, the alternative SWB measures from the KLPS are also distributed around zero (with negative and positive effects at every follow-up; see Appendix A3.1). This pattern of results is consistent with a very small or non-existent effect.
- A statistically non-significant effect does not prove the null hypothesis (i.e., that the effect is zero), but we can use Bayes factors to convey the probability that the effects of deworming are zero (Wagenmakers et al., 2010). Bayes factors compare the probability of hypotheses within the prior belief versus within the posterior belief (the new belief once the data is included with the prior belief). Using Bayes factors, we calculate that, if you come to this data with a weak (very uncertain) prior view of the effectiveness of deworming, then you will believe that the odds of the initial effect being zero (rather than not zero) are much more likely (4 to 15 times) after incorporating this evidence. We expand on this technical topic in Appendix A4.
- Comparing Group 1 to Group 2 (instead of Groups 1 and 2 to Group 3) finds a non-significant, negative initial effect which becomes (non-significantly) positive over time. We don’t have good reasons to believe this pattern of effects and it seems problematic for deworming that Group 1 which receives 6 years of deworming would fare worse than Group 2 which receives 5 years of deworming. This reinforces our belief that there is no effect on happiness from deworming in this data. See Appendix A3.2 for more details.
- We can see if these effects are meaningful by running a cost-effectiveness analysis to see if the per dollar effect is large enough to be cost-effective relative to other interventions we’ve reviewed. But as we show in the next section, without making some very strong assumptions, the total effect and cost-effectiveness of deworming also look null.
- We also find null results using other statistical approaches. In our primary analysis, we use a meta-regression, which calculates the effects independently at each time point using summary statistics (see Section 2). In a robustness test, we also analysed the individual-level data using a linear mixed effects model, which can produce more precise estimates when participants complete surveys multiple times or have missing data. Using this method, we also find small, non-significant results. The results also predict that the effects will reach zero and turn negative by around 5-6 years. We prefer the meta-regression model because it is consistent with our prior cost-effectiveness analyses for other interventions, the estimated effects are easier to convert to WELLBYs, and the results are more interpretable. See Appendix A3.3 for more details.
- While we find this null effect somewhat surprising if we take the income results of the KLPS seriously18When we convert GiveWell’s estimate of the economic benefits of deworming into WELLBYs, we find that deworming produces 28 WELLBYs per $1,000 donated. This is sizable but still 3 times less cost-effective than StrongMinds. See Appendix A3.4 for more details., it is less surprising if we consider how mixed the general literature is. If deworming did have an effect, it would be unclear what causal story explains the results. For example, if deworming really did make people richer, then you’d expect them to be happier too. The literature (see Section 1.3) yields many non-significant results for the short- and long-term outcomes of health, cognition, and education – so it’s unclear by what channel SWB would be improved.
3. Cost-effectiveness analyses
As mentioned in the previous section, we can use cost-effectiveness analyses to determine whether small effects are large on a per dollar basis. In this section, we explore how cost-effective deworming is based on our model. We believe our cost-effectiveness methods are relatively uncontroversial and we explain the process in detail in Appendix A1.19The steps include: getting the total effect; annualising the effects; extrapolating the effect from the PSDP context to the context of the charities by adjusting for worm burden, costs, and household size; adding speculative household spillovers; and getting the cost-effectiveness ratio. In this section, we only discuss the controversial part: how we calculate the total effect (i.e., the effect integrated over time) of deworming treatment. After that, we present the resulting cost-effectiveness. We also consider more speculative models of the cost-effectiveness.
3.1 A ‘face value’ cost-effectiveness model
We calculate the total effect by integrating the estimated initial effect (0.04 SDs) over time, while assuming the effect decays by -0.008 SDs each year. However, this requires us to decide when the effect begins and ends. These decisions are not straightforward, due to the following issues:
- When does the effect start? The average follow-up time of the responses from the first KLPS survey is mid-2004. However, deworming treatment for all groups ended – and data collection started – during 2003. It is unclear which precise point to use for the start of the effect.
- Recipients received deworming treatment for up to six years before the intervention. It is plausible they experienced a benefit during that time. However, there is no data for the wellbeing effect during that time. Do we implicitly assume the short-term benefit is zero, which may seems like an unreasonable assumption, or do we estimate the benefit in a completely speculatory manner?
- The effect of deworming also has an unclear duration. Normally, we would assume that an intervention’s benefit will decay until it reaches zero. However, two out of the three follow-ups to the KLPS show deworming has negative effects.20We did not have to address this issue in our previous analyses of psychotherapy and cash transfers because the initial effects and decay rates were statistically significant, and it was clear that there were no negative effects. This made estimating the total effect straightforward: the total effect was essentially the area of a triangle with its height at the initial effect and its base at the estimated duration that the intervention’s benefits lasted (i.e., until the effect reached zero). It seemed implausible that the benefits of psychotherapy and cash transfers decayed over time into harm, given that there were very few negative effect sizes (none of which were statistically significant) and many statistically significant positive effects. It doesn’t seem sensible to ignore most of the data by preventing the integration from turning negative – at least, absent some compelling causal story that we lack.
We do not have strong prior beliefs about the effect of deworming, so we think the best approach is to model the data at ‘face value’ by treating seriously our meta-regression model and the positive and negative effects across the data collection period. In this ‘face value’ model, we define the start of the effect as the time that treatment ended (i.e., 2003) – which is consistent with our analyses of other programs – and we define the end of the effect as the latest individual response in the data (i.e., just before 2015). Thus, the total duration of the effect is 11.75 years. Integrating over a longer time period would involve speculation beyond the data, and integrating over a shorter time period (e.g., stopping when the effect reaches zero) would ignore the negative effects at KLPS 2 and 3. While it seems plausible that we are missing short-term benefits of deworming on happiness that occur before treatment ends, this would involve speculating over periods in which we have no data. In general, we prefer the simplest, least speculative model that fits with the data and we believe the ‘face value’ model matches that idea. However, changing the assumptions of the model can strongly affect the cost-effectiveness results.
We present the total effect of the ‘face value’ model based on the decisions made above (illustrated below in Figure 5). Notably, the size of the benefit (area above zero) is roughly comparable to the size of the harm (area below zero). This results in a small, negative total effect of -0.05 (95% CI21We obtain 95% confidence intervals by running 10,000 Monte Carlo simulations. For our simulations we use normal distributions with the parameter estimate as the mean and the standard error as the standard deviation. Monte Carlo simulations allow us to propagate uncertainty from the effects to the cost-effectiveness ratio. For more details on this method, see Appendix A5.: -1.35, 1.24) SD-years which converts to -0.11 (95% CI: -2.92, 2.69) WELLBYs.22An effect in SD-years is the number of standard deviation changes across the years (e.g., 1 SD-year can be a change of 1 SD over a year or two SDs over two years). Having effects in SDs is useful because the findings from different measures are all in the same units. This is the standard method for meta-regression. However, these findings are difficult to interpret. We convert our results to WELLBYs by treating WELLBYs as point changes on a 0-10 life satisfaction scale. If we know the typical standard deviation on such a scale, then we can make the conversion. We use a conversion rate of 2.17, which is an average of the typical standard deviations found in the literature. So 0.11 SD-years * 2.17 = 0.24 WELLBYs. This reinforces our belief that there is no effect.
Figure 5: The total effect if we integrate over the data but no further
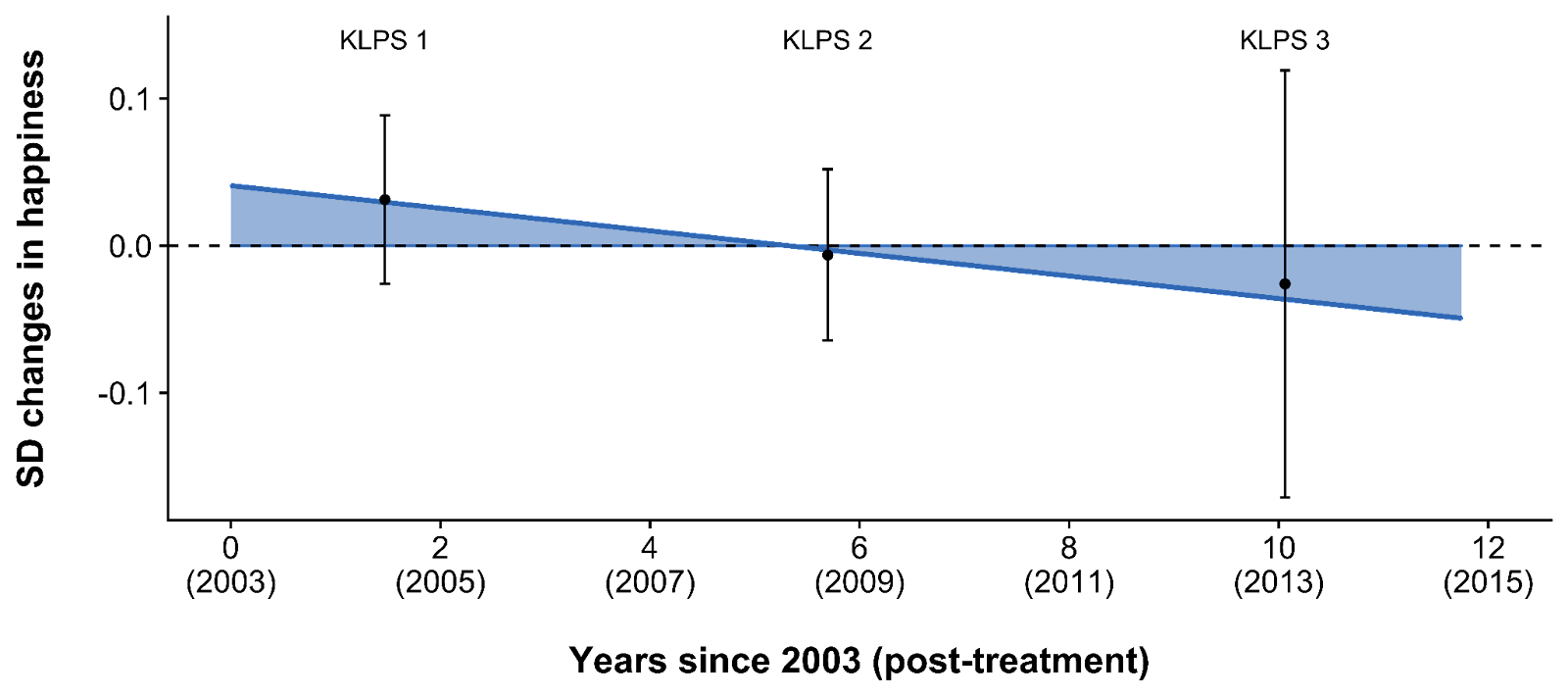
Note. The point estimates show the difference (in Cohen’s d) in happiness between the treatment and control group at each time point. The bars represent 95% confidence intervals. The blue area is the integrated total effect.
The resulting total effect is overall negative, which results in a negative cost-effectiveness estimate of -18 (95% CI: -613, 436) WELLBYs per $1,000 donated to Deworm the World (one of the four charities). Thus, the expected value shows that deworming is not as cost-effective as StrongMinds (77 WELLBYs per $1,000). This -18 WELLBYs looks like a large negative effect, but this is driven by the small costs of deworming: even small effects, when paired with low costs, can lead to large negative or positive cost-effectiveness estimates. The confidence intervals (built from Monte Carlo simulations; see Appendix A5 for more details), show that the cost-effectiveness of Deworm the World is incredibly uncertain – unsurprisingly because it incorporates imprecise inputs – to the point we consider it practically uninformative. Whatever prior view one held, it doesn’t seem that this evidence should update that view. As mentioned in Section 2.3, this cost-effectiveness analysis converges with our belief that there is no meaningful effect of deworming on long-term wellbeing. We illustrate the uncertainty around the cost-effectiveness of Deworm the World alone in Figure 6, and jointly with the other charities we’ve reviewed (which are all much less uncertain) in Figure 7.
Figure 6: Cost-effectiveness distribution of Deworm the World
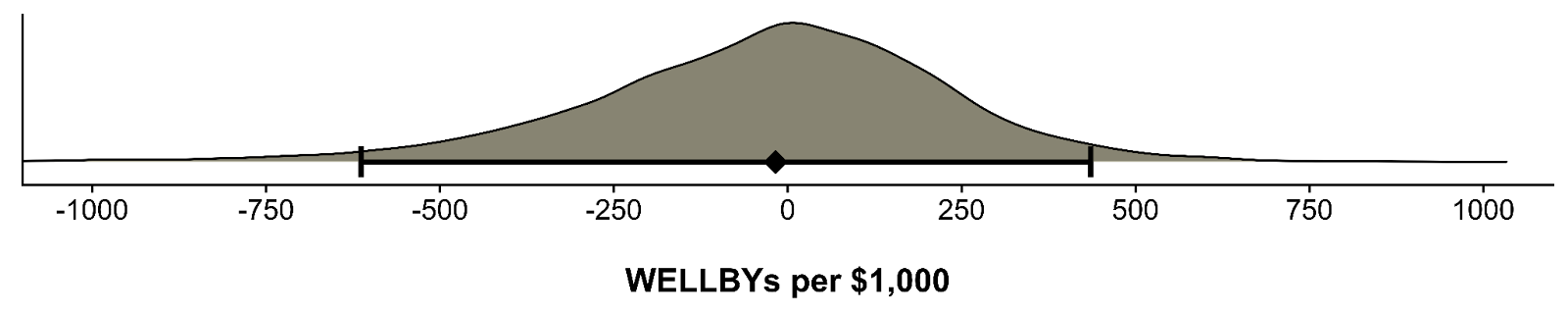
Note. The density plot shows the results of Monte Carlo simulations which estimate the uncertainty distributions. The distribution is skewed because the simulation contains cost estimates close to zero, resulting in a few large negative and positive cost-effectiveness figures.
Figure 7: Cost-effectiveness distributions of Deworm the World, GiveDirectly, and StrongMinds
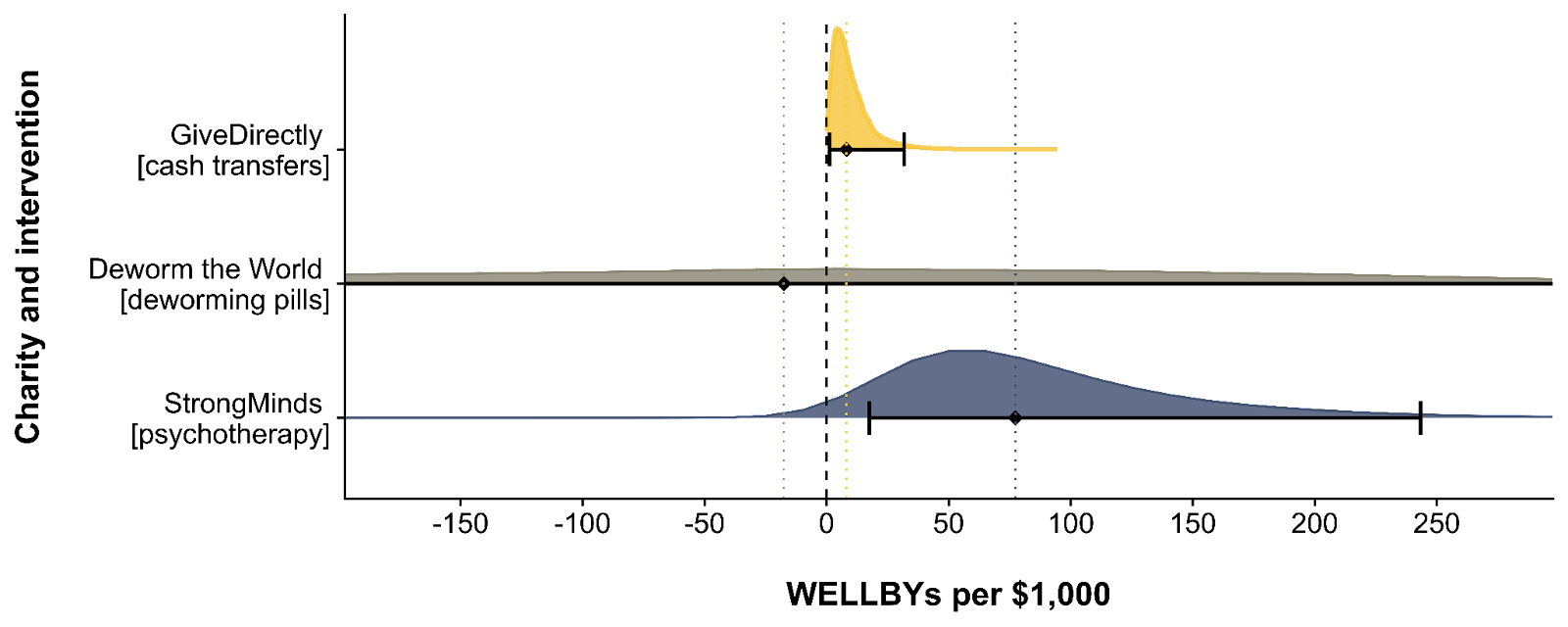
Note. The dashed line represents zero. The dotted lines represent the point estimates of the cost-effectiveness of the different charities. The density plots show the results of Monte Carlo simulations which estimate the uncertainty distributions. The distributions are skewed because the simulation contains cost estimates close to zero, resulting in a few large cost-effectiveness figures. The Deworm the World distribution appears less skewed because a tiny cost can lead to both large positive and negative cost-effectiveness figures, and because its probability density is much more spread out. The distribution of Deworm the World is cropped because it is extremely wide and uncertain.
3.2 Alternative specifications
The negative effect sizes may strike some as implausible, but we don’t think that this is obvious. There are tangible reasons that deworming could cause harm (see GiveWell’s section on the potential negative effects of mass deworming). An in-depth analysis of these reasons is beyond the scope of this report, but the ones mentioned in GiveWell’s report that seem the most important to us are: disrupting routine healthcare, side effects of drugs, and increasing the risk of malaria infections (the process by which this might occur is unclear).
Nevertheless, some readers might have strong beliefs that there are no negative effects and that effects at KLPS 2 and KLPS 3 are just zero. To us, it seems unacceptably ad hoc to take the positive effect at face value but discard the negative evidence entirely. Nevertheless, we present an ‘optimistic’ (but still constrained) model choice for such readers (see Appendix A1), which suggests that Deworm the World produces 39 (95% CI: -149, 188) WELLBYs per $1,000 donated. Hence, StrongMinds is 2 times more cost-effective than the ‘optimistic’ model.
Another concern could be that we are not considering potential short-term benefits between 1998 and 2003. There is no SWB data that we know of for this period, so any modelling choice adding those effects would be extremely speculative. We present a range of possible speculative modelling choices in Appendix A2. Averaging all of these together (we’re unsure if this approach is defensible – we do it for simplicity) we find that the models result in a cost-effectiveness of 31 WELLBYs per $1,000. Hence, StrongMinds is still 2.5 times more cost-effective.
4. Our recommendation for donors
We recommend charities that meet both of the following two conditions:
- There is strong evidence for the effectiveness of the intervention.23We have not yet formalised our criteria for “strong evidence”, but we take into account factors like the extent of the evidence (the number of studies and number of participants), the rigour of the research methods, and the execution of the studies, and the precision of the effect size estimates.
- The charity is more cost-effective than the best charity we’ve found so far. At the time of writing, our recommended charity is StrongMinds which generates 77 WELLBYs per $1,000 (McGuire et al., 2022b).
According to our analysis of the KLPS data, deworming charities do not satisfy either condition.
As we mentioned in Section 2.3, there are several reasons why we interpret the KLPS results as indicating that deworming has no effect. The pattern of the results (tiny positive and negative effects) and alternative analyses (Bayes Factors, comparing Groups 1 and 2, and an individual-level analysis) provide converging evidence that there is no meaningful effect of deworming on happiness in the KLPS.
Despite the uncertainty of the effect, we examined how cost-effective deworming would be if we took our model and the data seriously (see Section 3). This also suggests that there is no effect of deworming on happiness in the KLPS. Our other methods for calculating the cost-effectiveness (which are all more speculative) also suggest that deworming is less cost-effective than StrongMinds (see Appendix A1 and A2). This increases our confidence in abstaining from recommending deworming.
Even if our cost-effectiveness model had concluded that Deworm the World is as cost-effective as the best charity we’ve found so far – StrongMinds – we would be extremely hesitant to recommend it. Our general prior is that most interventions are less cost-effective than giving people cash (although we haven’t defended this formally). Ideally, our cost-effectiveness analyses rely on large meta-analyses of independent, rigorous studies. In this case, the available data comes from a single study with a number of limitations (see Section 5). Furthermore, the broader literature on deworming (see Section 1.3) is filled with mixed results and fails to provide a consistent causal story to inform our understanding of how deworming might improve wellbeing. Thus, to shift our prior, we would want to see more rigorous evidence documenting the benefits of deworming on subjective wellbeing.
Although we do not recommend deworming at this time, more evidence could change our minds in the future. When the effects of an intervention are so uncertain, and based on a single study, it is relatively easier for new evidence to shift our view.
5. Limitations
Our analysis of the KLPS data has several limitations that make us uncertain about the results, in rough order of importance:
- Our analysis is based on data from a single study, the KLPS. We have much greater confidence when effects are replicated in multiple studies, ideally randomised controlled trials with preregistered analysis plans. The lack of additional evidence makes us very uncertain about these results. Furthermore, more research tends to reduce effect sizes (e.g., because of publication bias), so there might be some bias in relying on only one study.
- The follow-up data were collected years after deworming treatment was received, and only after both the treatment and control groups had received some treatment. As a result, we do not know if there are short-term effects of deworming on SWB, which means we may be underestimating the effects of deworming (if they exist). However, as we discussed in Section 1, the evidence of other short-term effects on health and education is mixed and widely debated, so it is unclear if short-term effects should be expected.
- The KLPS data does not include a high-quality measure of SWB, which could make our results less reliable. The happy123 question is face valid, but measures with fewer than five options are not optimally reliable (Krosnik, 2009). As discussed in Appendix A3.1, we attempted to address this by including all the measures of SWB into a single model and found similar results. However, it would be ideal to have a measure that includes multiple items capturing happiness and life satisfaction, on a 0-10 scale where the endpoints afford clear socially comparable anchors (i.e., “(0) 10 indicates as extremely (dis)satisfied as a human can reasonably be”).
- The PDSP does not contain a strict control group (i.e., none of the groups received no deworming), so we do not have a clear estimate of the difference between no deworming and some deworming. It is possible that this comparison could show that deworming has a stronger effect if additional deworming has diminishing returns to wellbeing.
6. Future research to address limitations
We think the best way to address the limitations of the KLPS data is to find or collect more evidence of the impact of deworming on wellbeing. If no wellbeing data can be obtained, an alternative approach could model the effects of deworming on wellbeing via other outcomes (such as income or mortality and fertility effects). A further topic of research is to improve how we adjust for potential bias from relying on a single study. We discuss each of these approaches in more detail below.
6.1 Including more data
We see two ways to incorporate new data into our analysis.
First, we would be most excited to see new experimental studies that include high-quality SWB outcome measures.24There’s another follow-up to the PDSP study, the KLPS 4, which is expected to become available in the next year. This data will provide more insight into the long-term effects of deworming, but we don’t expect it will add much more clarity to our analysis. The KLPS 4 will have the same limitations as the current data and, if it had a large (statistically significant) effect on SWB, that would be hard to square with the rest of the data from the study. We recommend using a SWB measure with the same 0-10 scale over multiple follow-ups, and measuring potential spillovers on the household and community. These studies do not need to be long-term. Collecting SWB data from the very beginning of the deworming process or even before the start of treatment could provide more insight into the short-term impacts of deworming and greater power to detect small benefits from treatment.
Second, it is possible that the historical quasi-experimental analyses of the lifetime impact of deworming eradication – those done by Liu and Liu (2019), Bleakley (2007), and Makamu et al. (2018) – could be extended to include SWB as an outcome. These studies combined data from different sources across time, and it might be possible to match their data with panel surveys that contain SWB. We haven’t looked into this yet.
6.2 Including more outcomes (and converting them to WELLBYs)
Since we started writing this report, new research came out indicating that deworming in the PDSP significantly reduced mortality for the children of the recipients of deworming (Walker et al., 2022). We haven’t had time to review this work in sufficient detail to incorporate it into this report which only considers the life-improving effect of deworming. Extending life could generate WELLBYs but mortality effects are complex to model. At the technical level, we would have to model how fertility and mortality rates interact. Additionally, the value of life extension depends on difficult philosophical questions – a topic we discuss in depth in Plant et al. (2022). With this comes an additional layer of philosophical questions about saving the lives of people who do not yet exist (i.e., the next generation).
In the absence of more wellbeing data, one could indirectly estimate the life-improving effects of deworming on wellbeing through intermediate pathways such as by looking at how deworming affects education and then estimating how that change would affect wellbeing. We illustrated these potential pathways in Figure 3 in Section 1.3.4. However, we have not pursued this approach because the effects on these pathways are uncertain (each pathway is heavily debated, as we presented in Section 1.3) and conducting this type of research is time-consuming.25This would require four analyses to estimate the effect of deworming on health, cognitive abilities, income and education, and then four more to estimate the effect of health, cognitive ability, income and education on wellbeing. Not counting the mediating pathways such as deworming -> cognitive -> income -> wellbeing.
6.3 Correcting for bias from limited data
The analysis of deworming raises two general issues with estimating the cost-effectiveness of very cheap interventions with small and highly uncertain effects.
The first issue is that it is very expensive to research interventions with small effects because they require much more data to distinguish their effects from zero.
The second issue is that it’s better to rely on multiple studies than a single study because more studies make the estimates more precise, but also because replication studies tend to find much smaller effect sizes (e.g., Klein et al., 2018).26For example, large, multi-lab replications of classical psychological science findings led to lower effect sizes 75% of the time, where, on average, the effect sizes were 1 – (0.15/0.60) = 75% smaller after replication (Klein et al., 2018). Given the latter consideration, it is plausible to imagine discounting our cost-effectiveness figures for deworming – because they come from only one study – to address some of this tension.27For example, GiveWell discounts their estimates of the economic benefits of deworming by 87%. Our understanding is that GiveWell applies this replicability adjustment to the results so they align with their prior that the effects are much smaller. They determine the size of the adjustment based on a combination of subjective and empirical approaches (see here and here for details). See also SoGive’s exploration of this adjustment. We have not yet formed a principled approach to applying replicability adjustments. In this case, we have already concluded that the data are too uncertain to recommend deworming, so any additional adjustments would only reinforce our conclusion. However, we think that more research on this topic is important.
7. Conclusion
As far as we know, this is the first analysis of the effects of deworming on subjective wellbeing. Using long-term follow-up data from the KLPS, we found that deworming had small, non-significant effects on subjective wellbeing, leading us to conclude the effect of deworming is either non-existent or too small to estimate with certainty. We found converging evidence of a null effect across a variety of robustness tests and in a cost-effectiveness analysis. These null results are also consistent with the literature on the effects of deworming on other outcomes, which consists of mixed and uncertain findings. Even with more speculative cost-effectiveness analyses that were generous to deworming, we found that deworming charities were still less cost-effective than StrongMinds (our current top charity recommendation). Taking all this evidence together, we do not recommend deworming charities. The most important next step for proponents of deworming would be to find or collect more data on the effects of deworming on subjective wellbeing.